Artificial Intelligence for the analysis of Montelupo Majolica
This research project starts as part of the national PhD program Artificial Intelligence for Society, coordinated by the Department of Computer Science at the University of Pisa.
The analysis of ancient ceramics has a strong connection with computers and statistics. As early as the 1950s, these tools were recognized as valuable for handling large datasets, and pottery sherds are indeed found in abundance at most archaeological sites (Djindjian 2019).
In recent decades, rapid progress in digitization and computer applications has led to significant advances in pottery analysis (see Bickler 2021 and Karl et al. 2022 for an exhaustive survey). Neural-based computing, e.g., the one exploiting neural networks, has developed into an advanced approach for categorizing material culture, pottery sequences, and figurative systems, as well as for encoding chronological information. It uncovers connections between different groups and identifies sub-typologies that assist in determining relative chronologies (Ramazzotti 2020). Notably, machine learning has proven to be both time-saving and capable of achieving accuracy comparable to that of expert archaeologists in sorting and classifying large assemblages, while also uncovering meaningful patterns (Parisotto et al. 2022).
This research proposal aims to utilize deep learning techniques to enhance the value of a “territorial” asset such as the late and post medieval majolicae of Montelupo Fiorentino, which are distinguished by their extensive decorations.
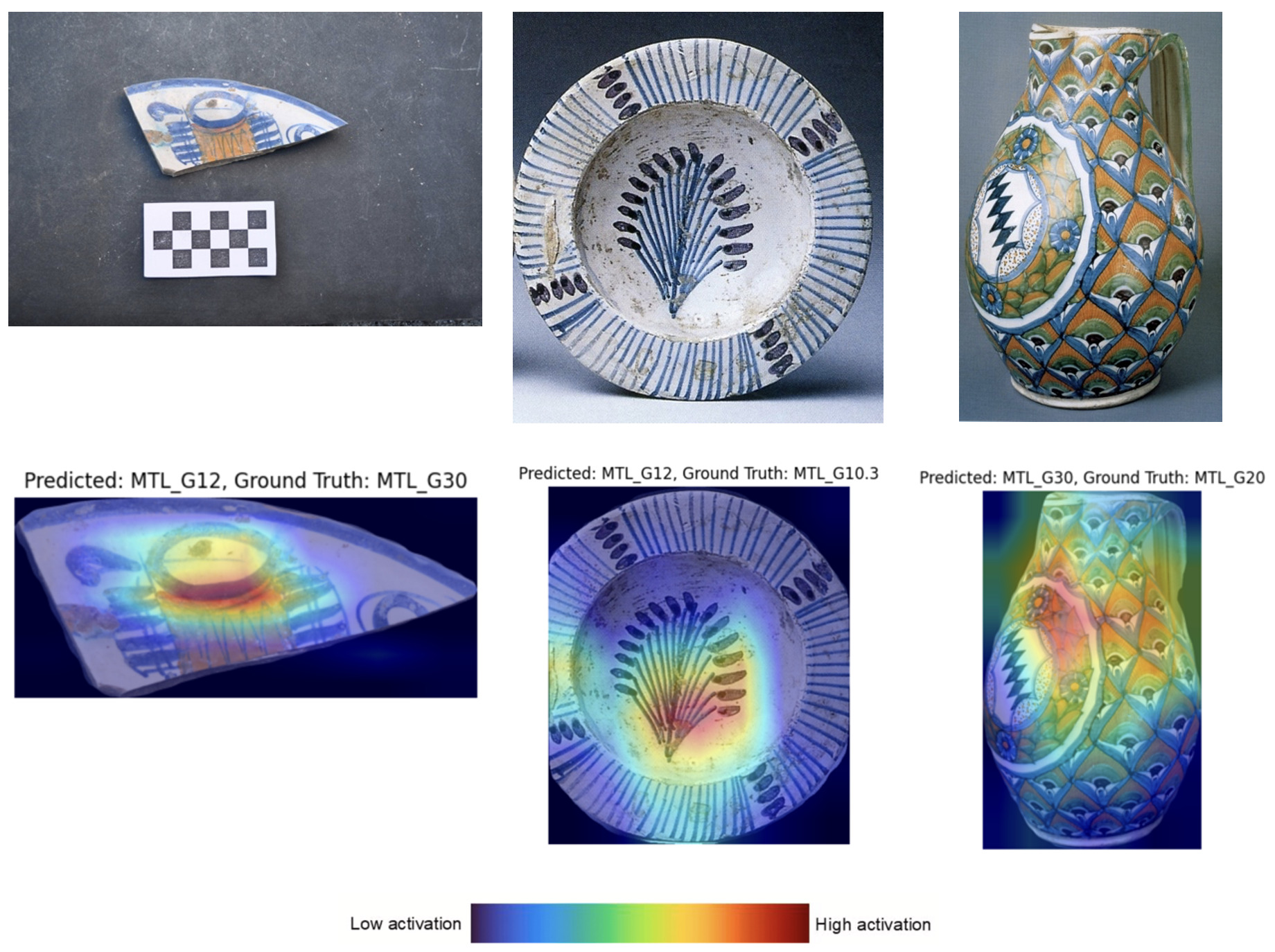
It is expected to move along two research lines:
- Efforts will focus on improving the classification based on the appearance of these ceramics, specifically working on those classes that, due to decorative details or low representativeness, are more challenging than others, this way trying to tackle some specific issues highlighted by ArchAIDE project. The so-called Explainable Artificial Intelligence (XAI) allows to visualize which part of the potsherd and its decoration the convolutional neural network focuses on to make its prediction. These “high activation” areas look red. By investigating further the model’s decision-making process, it is possible to understand why its prediction differs from the classification accepted by the scientific community (the “ground truth”).
- 3D models of complete informative and realistic pots will be created using Generative Artificial Intelligence techniques. They could be incorporated into virtual reconstructions, useful for museum exhibitions, educational purposes, or the entertainment industry, this way contributing to the field of digital restoration and preservation of these ceramics.
References
Bickler 2021
Simon H. Bickler. Machine learning arrives in archaeology. Advances in Archaeological Practice, 9(2):186–191, 2021.
Djindjian 2019
Franc ̧ois Djindjian. Archaeology and computers: a long story in the making of modern archaeology. Archeologia e Calcolatori, 30:13–20, 2019.
Karl et al. 2022
Stephan Karl, Peter Houska, Stefan Lengauer, Jessica Haring, Elisabeth Trinkl, and Reinhold Preiner. Advances in digital pottery analysis. it – Information Technology, 64(6):195–216, 2022.
Parisotto et al. 2022
Simone Parisotto, Ninetta Leone, Carola-Bibiane Sch ̈onlieb, and Alessandro Launaro. Unsupervised clustering of roman potsherds via variational autoencoders. Journal of Archaeological Science, 142:105598, 2022.
Ramazzotti 2020
Marco Ramazzotti. Modelling the past. logics, semantics and appli- cations of neural computing in archaeology. Archeologia e Calcolatori, 31:169–180, 2020.
Progetto a cura di:
Federica Mauro
federica.mauro@phd.unipi.it